lenet5 논문
https://yann.lecun.com/exdb/publis/pdf/lecun-01a.pdf
https://github.com/stonegyoung/Pytorch_Study/blob/master/lenet_%EA%B5%AC%ED%98%84%2BMNIST.ipynb
Pytorch_Study/lenet_구현+MNIST.ipynb at master · stonegyoung/Pytorch_Study
Pytorch 공부. Contribute to stonegyoung/Pytorch_Study development by creating an account on GitHub.
github.com
Lenet5 모델을 만들어보겠습니다.
필요 라이브러리 임포트
import torch
import torch.nn as nn
nn.Linear을 사용하는 정형 데이터와는 달리
이미지 데이터 처리에서는 nn.Conv2d를 사용합니다.
nn.Conv2d(
in_channels = 인풋 채널 수,
out_channels = 나오는 필터의 개수,
kernel_size = 필터(커널) 사이즈
)
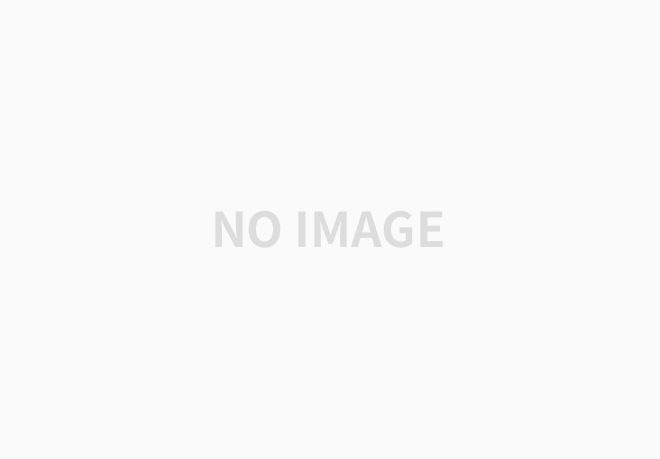
Lenet5 구조를 보겠습니다
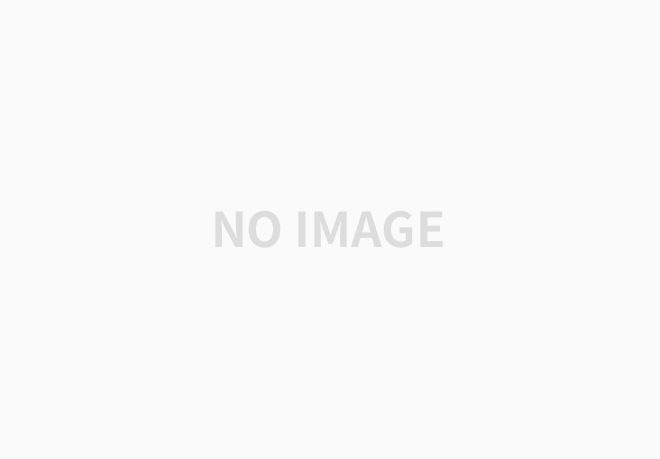
1. Conv1(C1)
Input : (1, 32, 32)
1 채널(흑백)의 32*32 사이즈의 이미지가 인풋으로 들어갑니다
Output : (6, 28, 28)
아웃풋으로 6개의 feature map이 나옵니다
Kernel : 5*5
stride : 1, padding : 0
Activation : Tanh
nn.Conv2d(in_channels=1, out_channels=6, kernel_size=5)
2. Polling1(S2)
Max-Pooling
Input: (6, 28, 28)
Output: (6, 14, 14)
Kernel: 2*2
stride: 2
nn.MaxPool(kernel_size=2)
3. Conv2(C3)
Input: (6, 14, 14)
Output: (16, 10, 10)
Kernel: 5*5
stride: 1, padding: 0
Activation: Tanh
4. Polling2(S4)
Max-Pooling
Input: (16, 10, 10)
Output: (16, 5, 5)
Kernel: 2*2
stride: 2
5. Conv3(C5)
Input: (16, 5, 5)
Output: (120, 1, 1)
Kernel: 5*5
stride: 1, padding: 0
Activation: Tanh
6. FC Layer(F6)
Input: 120
(120, 1, 1)을 1차로 쭉 펴는 Flatten
Output: 84
Activation: Tanh
7. Output Layer(OUTPUT)
Input: 84
Output: 10
클래스 수
Activation : X
이런 식으로 모델 구조를 class로 짜보겠습니다.
class Lenet(nn.Module):
def __init__(self):
super(Lenet, self).__init__()
# 처음 in_channels = 채널의 개수 = 1개(흑백)
# out_channels = 나오는 필터의 수
# 32*32 흑백 이미지와 5*5 필터 6개가 합성곱 연산 -> 28*28 크기의 특징맵 6개가 만들어진다.
self.c1 = nn.Conv2d(in_channels=1, out_channels=6, kernel_size=5)
# cnn도 활성화함수 필요함. lenet은 tanh 씀
self.act1 = nn.Tanh()
# 풀링(이미지 줄이기. 특징 수는 똑같) maxpooling = subsampling
self.s2 = nn.MaxPool2d(kernel_size=2)
# 14*14 특징맵 6개와 5*5 필터 16개가 합성곱 연산 -> 10*10 크기의 특징맵 16개가 만들어진다.
self.c3 = nn.Conv2d(in_channels=6,out_channels=16, kernel_size=5)
self.act2 = nn.Tanh()
self.s4 = nn.MaxPool2d(kernel_size=2)
# 5*5 특징맵 16개와 5*5 필터 120개가 합성곱 연산 -> 1*1 크기의 특징맵 120개가 만들어진다
self.c5 = nn.Conv2d(in_channels=16, out_channels=120, kernel_size=5)
self.act3 = nn.Tanh()
self.fc6 = nn.Linear(120, 84)
self.act4 = nn.Tanh()
self.output = nn.Linear(84,10)
def forward(self, x):
x = self.c1(x)
x = self.act1(x)
x = self.s2(x)
x = self.c3(x)
x = self.act2(x)
x = self.s4(x)
x = self.c5(x)
x = self.act3(x)
x = x.view(-1, 120) # (-1, 120) * (120, 84) -> (-1, 84)
x = self.fc6(x)
x = self.act4(x)
x = self.output(x)
return x
model = Lenet()
model
잘 만들어졌는지 테스트하기
(channel, height, width)를 넣어본다
# test
data = torch.rand(1,32,32)
data.shape
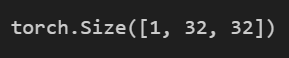
model에 이 값을 넣으면 (1,10) shape이 나오면 됩니다.
model(data).shape

(batch_size, channel, height, width) 넣어보기
data = torch.rand(32,1,32,32)
model(data).shape

'Pytorch' 카테고리의 다른 글
[파이토치] Custom Dataset 만들기 (0) | 2025.01.08 |
---|---|
[파이토치] CNN 모델 학습, 평가, 추론 (0) | 2025.01.06 |
[파이토치] MNIST로 이미지 데이터 처리 (1) | 2024.12.31 |
[파이토치] 이미지 데이터 전처리/증강 (1) | 2024.12.28 |
[파이토치] CNN (0) | 2024.12.26 |